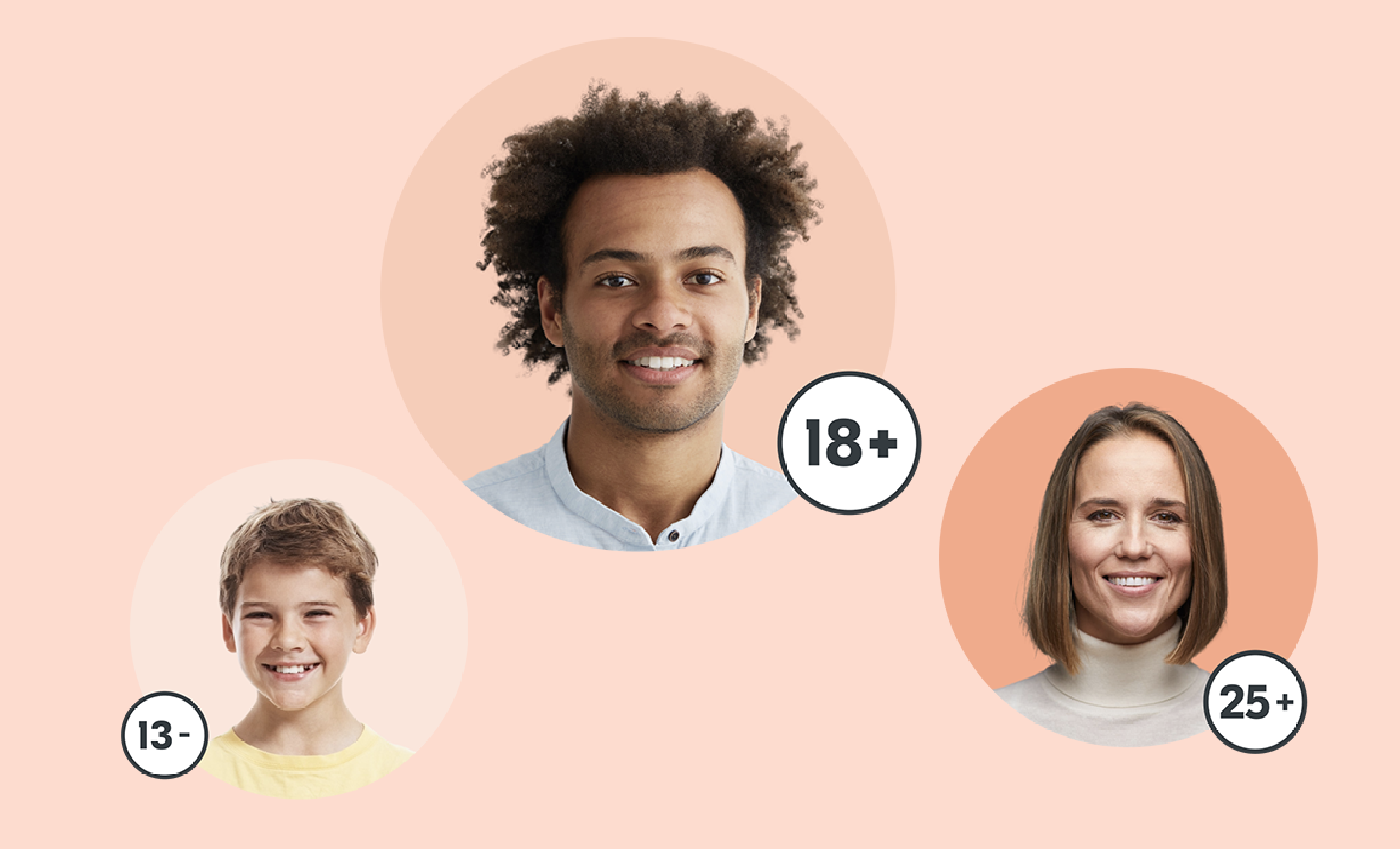
We continue to invest in improving the accuracy of our world-leading facial age estimation and we will soon be releasing a new ‘Jan 2023’ model with improved accuracy across all skin tones and gender for those aged 6-70.
At Yoti we constantly strive to improve all our services given our commitment to tech for good, and given the growing market importance of these services and increasing regulatory engagement. For example, coming soon we’ll be introducing multiple, concurrent age estimation models, which will help improve accuracy even further, whilst still completing a check within seconds.
We will also continue to update our Yoti Age Estimation white paper, now 4 years old this month, in which we transparently publish our accuracy across age, gender and skin tones by year between ages 6 and 70. We expect to publish the updated white paper in the next couple of weeks but importantly, we can share today our Jan 2023 model further improves our accuracy and further reduces bias.
Across all age ranges, gender and skin tones we are seeing a 5.1% increase in accuracy, reducing the weighted average MAE for 6 to 70 year olds from 3.0 to 2.8 years. As you can see below for this model we have been focussing on reducing the discrepancy between light skintones (tone 1) and darker skin tones (tone 3) We recognise our work is not finished in this regard.
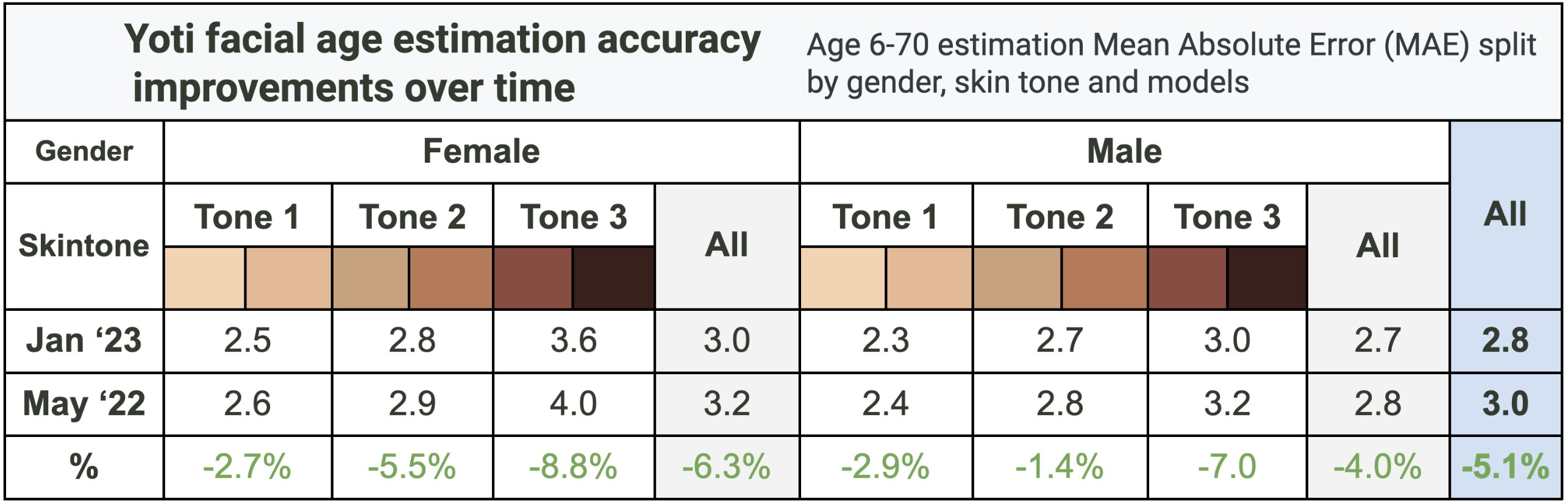
Table shows mean average error (MAE) for age range 6-70 by gender and skin tone, and the percentage improvement from our May 2022 model to our January 2023 model.
So, well done for marking your own homework, you might say! But we are happy to report our May 2022 white paper has been independently verified as to the measurement methodology and accuracy of our results. On the request of one of our clients, the ACCS undertook an independent evaluation of our May 2022 white paper and had this to say:
“The training, testing and results reporting presented in the whitepaper have been independently validated by ACCS, who have certified that Yoti have deployed appropriate methodologies to analyse the performance of their Age Estimation algorithm, including ensuring appropriate separation of machine learning training data, testing data and validation data.”
In addition to this, we are also happy to announce that we have been invited to participate in a workshop on ICO commissioned research on the measurement of age assurance on Thursday 19th January, in London organised by ACCS and AVPA.
Follow us on LinkedIn to stay abreast of Yoti news or get in touch to find out more.