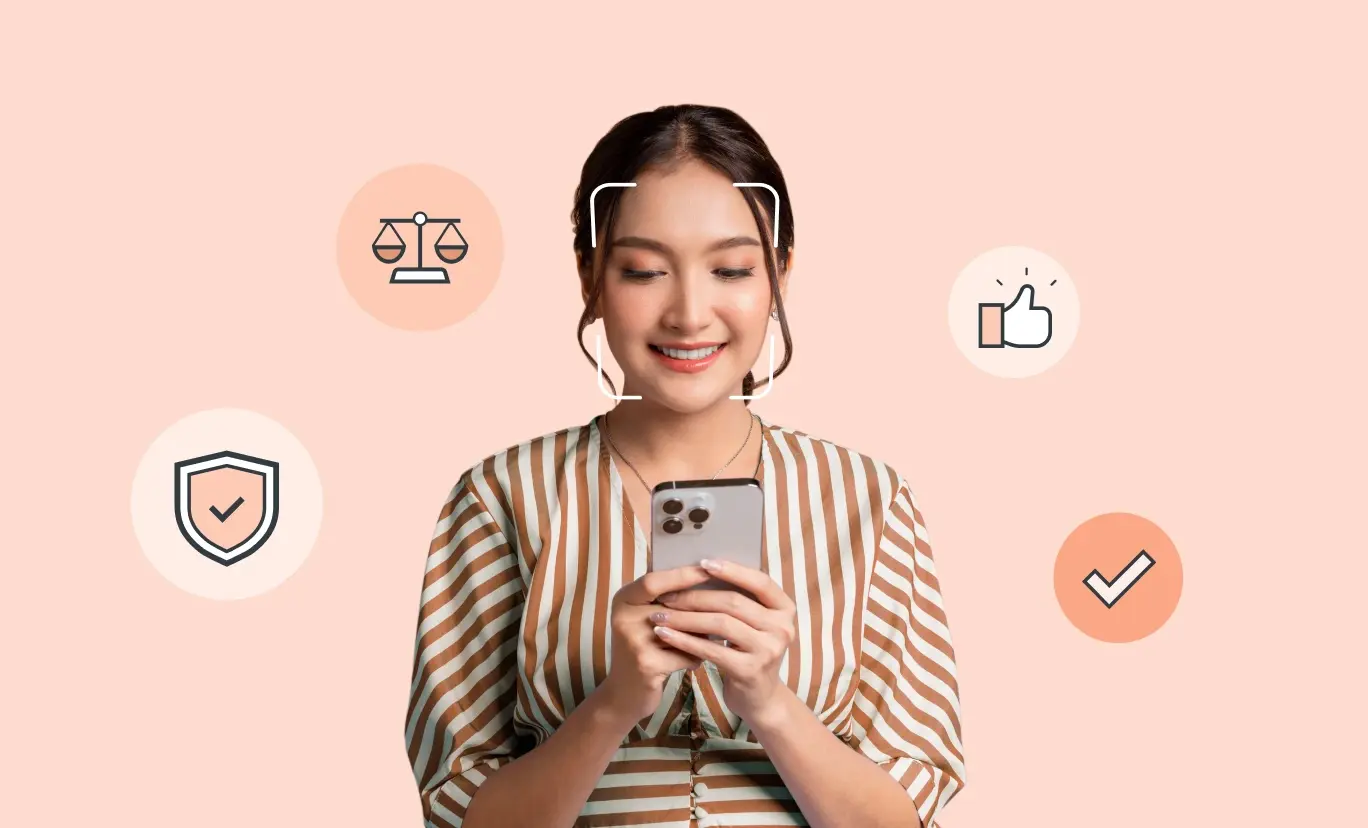
To protect young people online, businesses need to provide age-appropriate experiences for their users. This could apply to online marketplaces, social media networks, content sharing platforms and gaming sites.
But to put the correct measures in place, businesses need to know the ages of their customers. It was previously thought that the only way to confidently establish a user’s age was with an identity document, like a passport or driving licence, or checks to third-party databases such as credit reference agencies or mobile network operators. However, regulators are now recognising facial age estimation as an effective alternative.
As with any technology, transparency is key. We believe that businesses providing facial age estimation should be fully open with anyone who may use it. This helps to build trust, foster accountability and drive ethical development of the technology.
What is facial age estimation?
Facial age estimation determines a person’s age from a live facial image. It allows people to pass an age threshold in seconds without needing to share any personal details like their name or date of birth, identity documents or credit card details.
With high-quality liveness checks and injection attack detection, facial age estimation is a robust, effective and secure way of checking a user’s age. It doesn’t scan faces against a database and can’t recognise anyone, so it’s not facial recognition.
Why is transparency important?
When developing facial age estimation technology, transparency is crucial for several reasons. These include:
Legal compliance
Transparency can help businesses ensure that they comply with relevant legislation. These could be data privacy laws, non-discrimination laws and child protection laws. Our facial age estimation technology complies with both EU GDPR as well as our own ethical approach to user data and privacy.
To train the facial age estimation algorithm, we need to use images of faces that are associated with known ages. The majority of our training set comes from individuals who have added an identity document to their Yoti app. Individuals are informed about this use of their data (including their selfie image, month and year of birth and gender) before they add an identity document. And they’re able to opt-out of this processing at any time from within the app settings.
We believe the different accuracy rates shown for different groups (age, gender, skin tone) correlate strongly with how well-represented those groups are in the training data. Therefore, we sometimes require more data to improve how the technology works across specific demographics. In these cases, we perform data collection exercises by purchasing data from vetted suppliers. This is done with consent and in line with GDPR.
For example, all our training data for under 13s has been collected specifically for the purposes of training the facial age estimation model. It consists only of faces and month and year of birth. We ask parents and guardians to give consent during these specific data collection exercises. This additional data helped us to improve the accuracy of our technology for under 13s – a key age group that parents and regulators are interested in protecting online.
The UK’s Information Commissioner’s Office has confirmed that our facial age estimation does not involve the processing of special category data. Our facial age estimation model is unable to allow or confirm the unique identification of a person, simply because it’s not been trained to do so. This means that it’s not being used for the purpose of unique identification – the key test for special category data.
Ethical considerations
Alongside legal compliance, transparency also allows businesses to consider ethical issues. Ethics can guide how the technology is developed and used. It can encompass a range of factors including promoting fairness, protecting privacy and upholding societal values.
For us, transparency helps us to develop our facial age estimation technology in line with our ethics and principles. It allows both internal and external parties to help guide us in the right direction.
As we built our facial age estimation, we consulted several organisations such as the Center for Democracy & Technology and the World Privacy Forum. We’ve also invited scrutiny by hosting a number of roundtables with external bodies. Attendees included regulators; NGOs dealing with child safety, data and AI; universities and representatives from global platforms and consultancies.
We’ve also reviewed the positive, negative, intended and unintended consequences using the Consequence Scanning approach. And our independent Guardian Council regularly reviews our research, product development and deployment.
Input from these groups allows us to consider and address potential issues with the technology as early in the process as possible.
Bias mitigation
Transparency can help identify and address bias in facial age estimation technology. Algorithms which haven’t been effectively trained can produce inaccurate, unreliable or flawed results. This can lead to doubts about the fairness, equality and the ethical use of the technology.
Facial age estimation systems need to be trained using data which is known to be true, known as the ‘ground truth’. In our case, this data is the image of a face and the month and year of birth of the person in the image. This data is used to refine the algorithm – a set of rules followed by the technology to perform its calculations and deliver a result. As the algorithm is trained with more correct data, it “learns” to correspond the data with the correct results.
Sometimes, the technology may deliver less accurate results if it hasn’t been trained with as much data from certain demographic groups. If it consistently presents significant differences between users of different demographics, the system is considered to have bias. Where there are discrepancies, the algorithm should be trained with more data from the relevant dataset.
To know how accurate the technology is, it should be regularly tested. This testing should measure accuracy rates across a range of factors such as gender, skin tone and age. The technology should be tested both internally as well as by independent third-party organisations.
Until recently, there’s been no widely-recognised benchmark for facial age estimation algorithms. The National Institute of Standards and Technology (NIST) has now begun a global benchmarking programme. Its goal is to give regulators and relying parties confidence in the efficacy and bias levels of facial age estimation technology.
We believe that these accuracy rates should be made publicly available. That’s why we regularly publish our testing results in our white papers. By doing this, businesses and users are fully informed about how our technology works and how it’s progressing. This allows them to make an informed decision about whether they want to use the technology and if it’s suitable for their desired use case.
Building trust
When businesses are transparent about their facial age estimation technology, they can be held accountable for their actions and decisions. This should result in customers and users being more likely to trust them since they’re upfront about their operations.
For example, by publishing accuracy rates, businesses can demonstrate openness with their stakeholders. Stakeholders can include customers, clients and the wider community. By choosing to be honest about the limitations of their technology, businesses are more likely to work on improving it. In our case, since this data is available on our website to everyone, we’re held accountable publicly.
Embracing transparency in facial age estimation
The importance of transparency when it comes to facial age estimation cannot be understated. We believe that being open about how our technology works can lead to stronger relationships with stakeholders, built on trust.
If you’d like to know more about our facial age estimation technology, please get in touch.