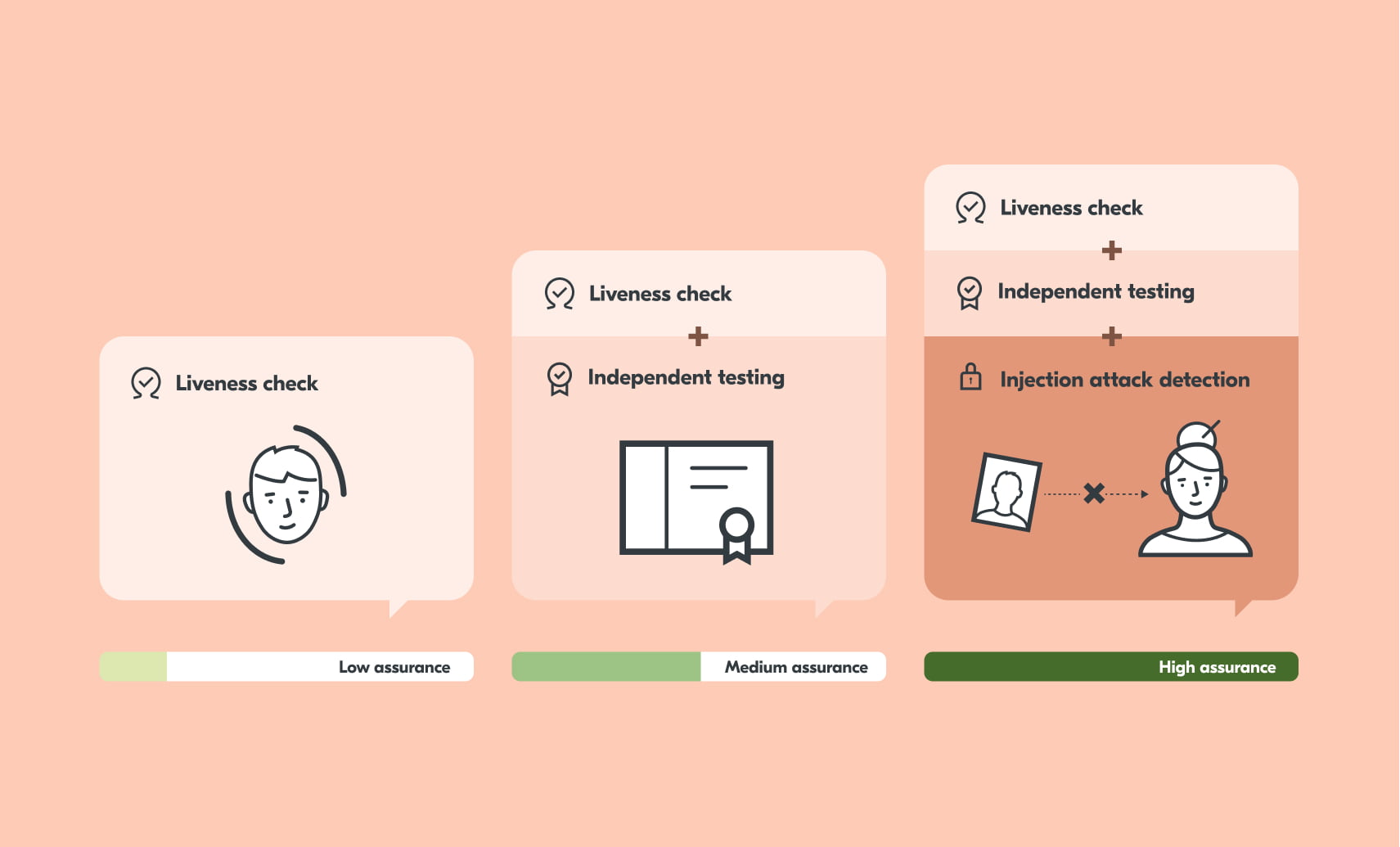
For platforms to deliver age-appropriate content, they need to know the age of their users. Age estimation technology can provide an inclusive and accessible solution. It’s possible to estimate a person’s age from a number of features, including their voice, face, palm or fingerprints.
Some age estimation methods are very accurate. Others collect little or even no personal data. But very few can do both.
When done to a high standard, effective facial age estimation can offer a high level of privacy and a high level of assurance.
Age estimation in the real world
Consider the following situation: a person who is clearly underage tries to buy alcohol from a supermarket.
When asked to prove their age, they present an identity document stating they’re over 18. If the cashier thinks the person looks under 18, they would reject the document. They would trust their instincts that the document may be fake, tampered with or belongs to someone else.
This ‘common-sense’ approach can be thought of as human age estimation.
Human age estimation
Through our experiences, humans have developed an ability to assess someone’s age. Over time we learn “that’s what people of a particular age look like”. We do this by observing visual cues such as wrinkles and hair colour.
But there are lots of reasons that can make it difficult for humans to correctly estimate another person’s age. We’re quite easily able to work out the ages of those closely aged to us. However, when we go outside of this range, our accuracy decreases. This accuracy also tends to fall as we ourselves get older.
It should be noted that various factors can affect the ageing process, such as:
- nutrition and quality of diet
- exposure to sun/wind
- use of narcotics
- cosmetic surgery
- physical labour
- stress
- lack of sleep
Retail staff often need to estimate the ages of customers who are buying age-restricted goods. But when viewing lots of faces in succession, a person’s judgement tends to be influenced by the faces they’ve just seen.
Some regulators have imposed safety buffers to allow for these challenges. For instance, the UK has implemented the Challenge 25 policy. If a person buying age-restricted goods looks under 25, they must present a physical ID to prove their age.
Facial age estimation
Facial age estimation has automated the age estimation process. It allows technology to estimate a person’s age. It is powered by an algorithm that’s learned to estimate age in the same way humans do – by looking at faces. The technology detects a live human face, analyses the pixels in the image and gives an age estimate.
Facial age estimation is not facial recognition; it estimates someone’s age without identifying them. It does so by converting the pixels of a facial image into numbers and comparing the pattern of numbers to patterns associated with known ages. It does not scan their face against a database or recognise anyone.
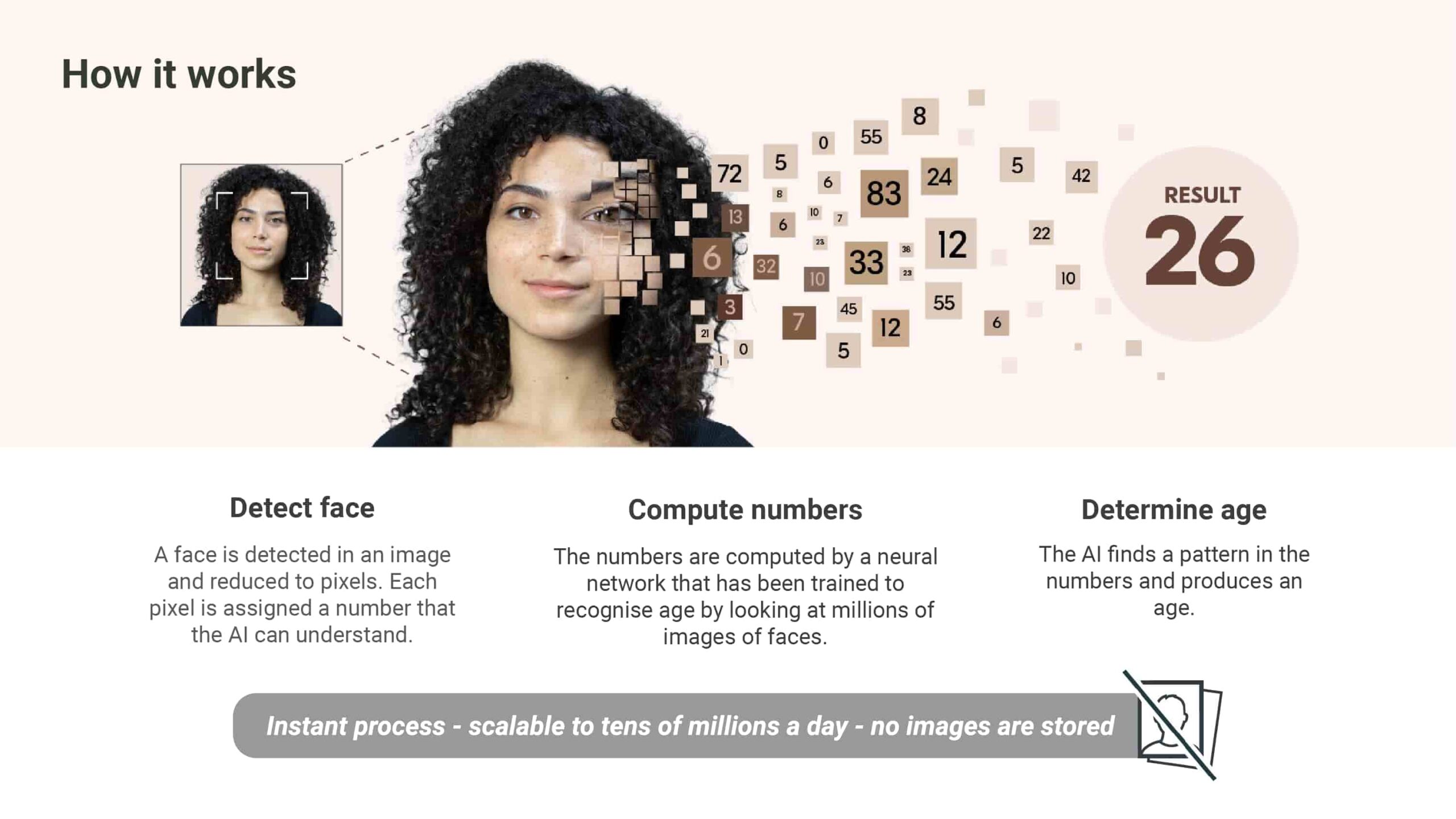
As soon as an age has been estimated, the facial image is immediately and permanently deleted.
Safety buffers
In certain sectors, it is illegal for someone under a specified age to access particular goods, services or experiences.
In these cases, companies can include a safety buffer when using facial age estimation. These account for potential errors and provide a necessary margin of safety. The size of this buffer can depend on the level of accuracy required by the business or on any regulatory requirements.
For example, if a business needs to know if someone is 18 or over, they might apply the Challenge 25 policy and set a seven-year buffer. This means that the technology must determine the user’s age to be at least 25.
What is effective facial age estimation?
Facial age estimation can give a high level of assurance when done effectively. Here are some of the steps required:
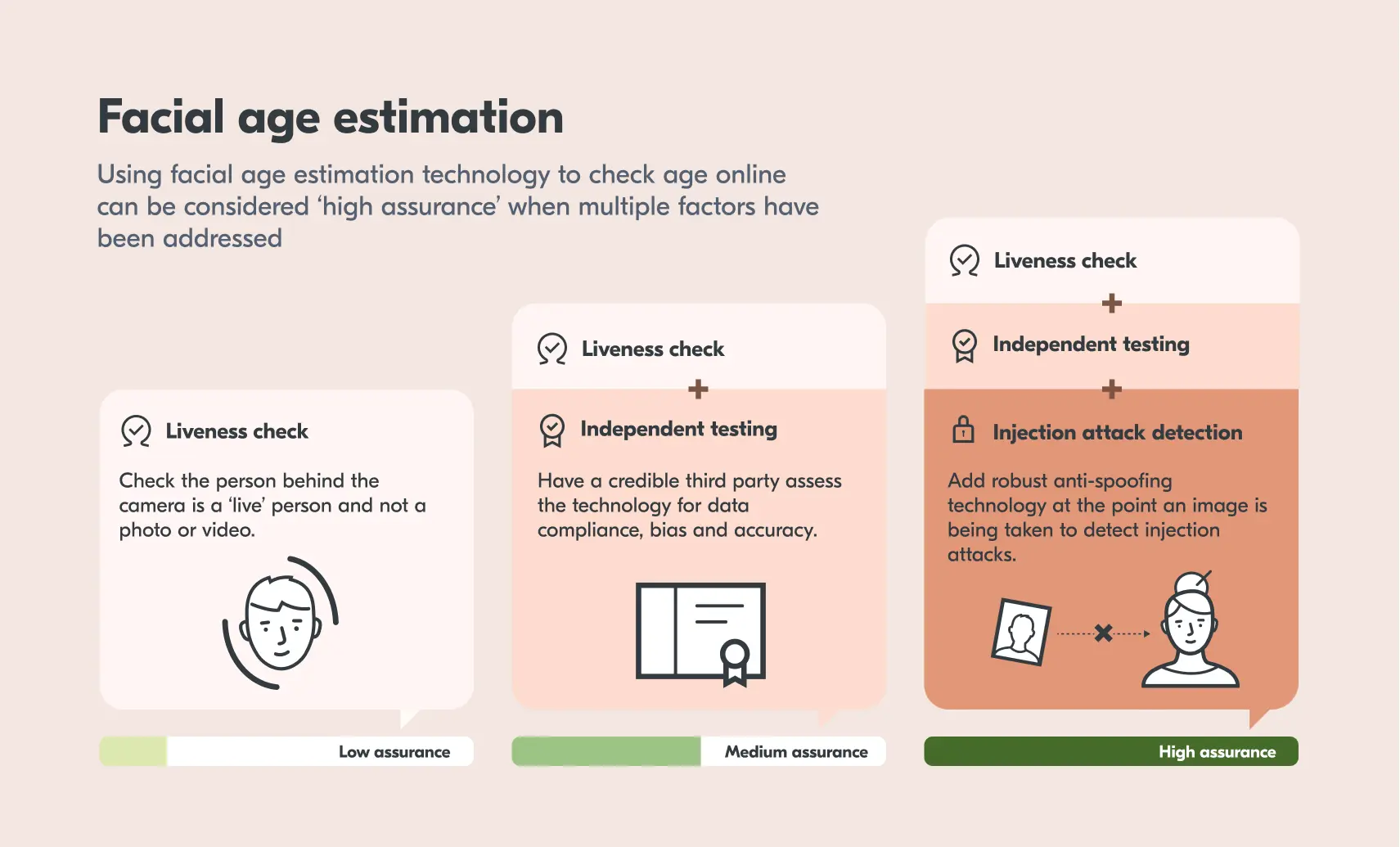
Liveness detection
Businesses need to be sure that the individual completing the age estimation is a real person. The technology must be able to detect whether the face shown is a live person, and not a photo, mask or video. Without this, a child could attempt to use a photo of their parent to pass the age check.
There are two types of liveness checks – active liveness and passive liveness. Active liveness checks require users to perform specific actions or follow some instructions. The user may have to nod their head or say certain words. Though effective, this may be difficult, particularly for users with disabilities.
Language, audio input and noisy environments can also be a problem for some users. Instructions could be misunderstood by those reading them in a second language. Or some people may be self-conscious if completing the liveness check in public.
Passive liveness works from a facial image. This creates a smooth, inclusive and more accessible user experience. The technology does not recognise any faces and does not save any images. It simply checks that the face in the image belongs to a real, live person.
NIST provides a framework for testing performance levels of liveness. A good benchmark for liveness is NIST Level 2. This involves testing against specialist attacks such as latex face masks or 3D printers.
Independent testing
With any technology, people can have questions about how it works and if it’s safe to use. Therefore, independent testing is a crucial part of effective facial age estimation. External accreditation is necessary for three main factors:
Data handling
Users need to be sure that their privacy is protected. They need to know that the technology does not recognise anyone, is not storing the image of their face, and that their data is being handled safely.
Facial age estimation providers need to deliver clear guidance on how the technology works. They must also be transparent about what happens with a user’s data.
Bias
Effective facial age estimation should be tested for bias. At a minimum, it will be assessed for its accuracy by gender, skin tone and each year of age. This is to ensure that the technology works fairly for all people. Where there are inconsistencies, efforts should be made to improve the algorithm.
Accuracy
The technology should also be assessed for accuracy. Alongside testing the algorithm, testing should be done in a live environment. For example, when used at a gambling machine in a bar, the technology needs to be able to adapt to different lighting.
The technology’s performance should be measured in terms of true positives, and false positives, success rates and completion times.
- If the model correctly estimates an 18-year-old to be under 25, this is a true positive.
- If the model incorrectly estimates an 18-year-old to be over 25, this is a false positive.
The aim is for a high true positive rate and a low false positive rate. This means that the right people can efficiently access certain age-restricted goods, products or experiences. And those who are outside of the correct age bracket are protected from accessing them.
It is good practice for age estimation providers to be transparent about these rates so that both businesses and individuals know how accurate the technology is.
Injection attacks
An injection attack involves injecting an image or video into the system. This is so the technology estimates the age of the face from the injected image, rather than the one in front of the camera
This is a particular issue for online facial age estimation where the image is taken with a laptop or mobile phone camera. With free software and limited technical ability, it’s fairly easy to swap an image for one that’s designed to pass the checks.
An effective facial age estimation system will be able to detect injection attacks. This is achieved with integrated anti-spoofing technology which can detect attacks at the point the image is taken.
So what does this all mean?
Facial age estimation can be an inclusive and proportionate method of age assurance. But the necessary controls must be in place to ensure that the technology delivers a high level of assurance and high privacy.
To find out more about how effective facial age estimation may be useful for your organisation, please get in touch.